How can we ensure the delivery of reliable ground truth to enable the development of safe autonomous vehicles?
The perception subsystem of an Autonomous vehicle (AV) must enable the overall vehicle to drive safely. Many car manufacturers and suppliers are considering the concept of Safety Element out of Context (SEooC) to be able to argue for a safe AV, basically assessing different elements in the AV as standalone components. In this context, one can talk about safe perception as a key component to be able to argue for a safe AV.
However, functional safety requirements on perception cannot be linked directly to ground truth, as there is no clear connection between individual mistakes and errors in ground truth and their effect on the evaluation of the perception performance or the training of a perception algorithm. While a small number of errors is unlikely to have a detrimental impact on perception system performance, in the end, using ground truth data with many errors and inconsistencies, especially systematic errors, will. At Kognic we consider data free of such issues to be reliable.
To make sure that Kognic is able to deliver such reliable ground truth, our platform needs to ensure that we deliver data that is free of systematic and random errors. Using terminology from HARA we have identified hazards in that endeavor, for instance:
- Annotation errors, e.g. objects are annotated incorrectly
- Incorrect data leaving our platform
When building the Kognic platform we take such hazards into account, so we can safely argue that the residual risk of systematic errors in data is low enough that our ground truth is reliable.
But how do we do this in practice?
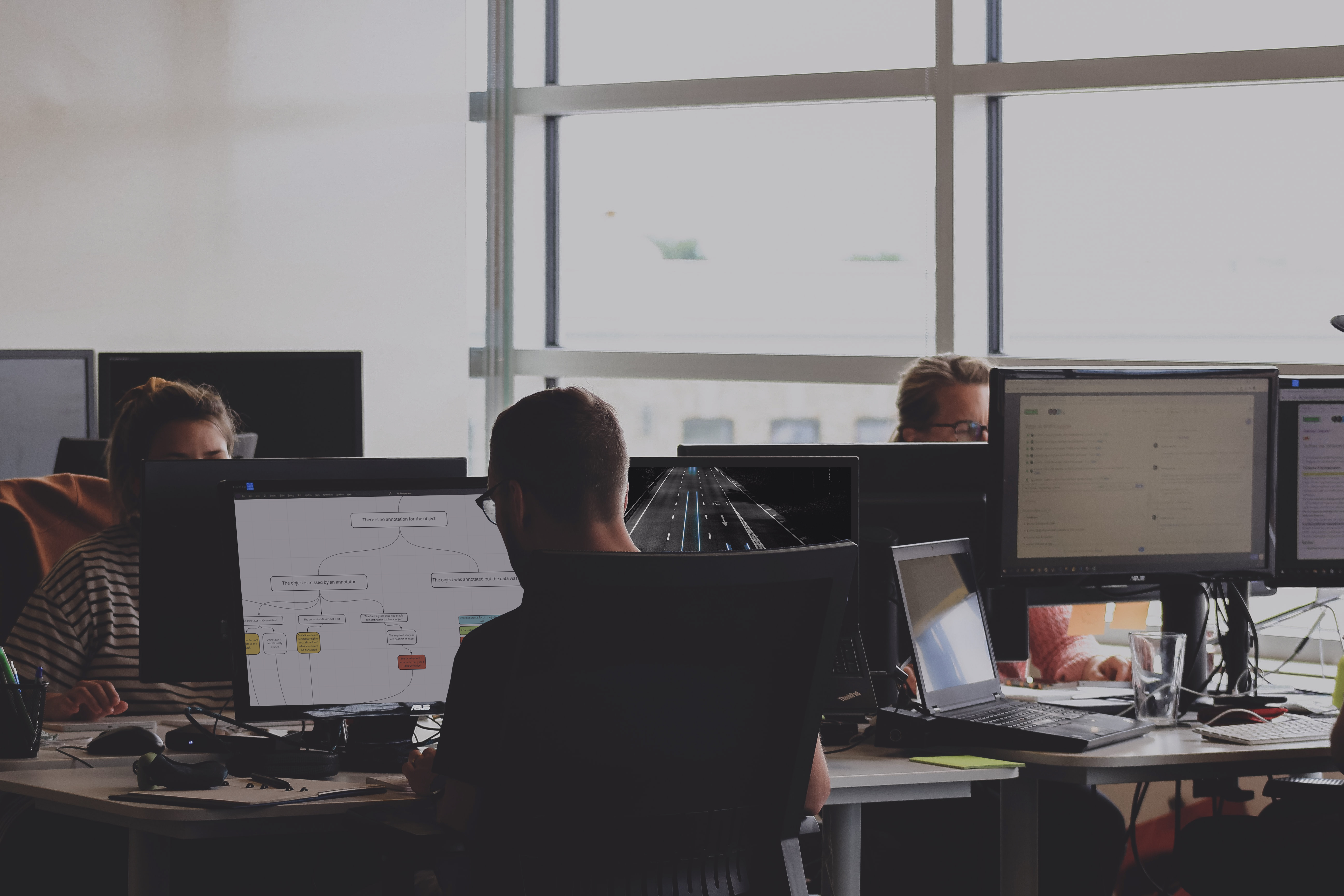
The first hazard above is related mainly to our ability to prevent errors when producing annotations. That means that mitigating these hazards is centered around having a reliable tool and ensuring annotator competence. The second hazard is related to our ability to discover incorrect annotations and prevent them from leaving our platform and that can be mitigated by having a sufficient quality assurance process.
To exemplify, consider a task where a number of images are to be annotated in order to train an object detection algorithm. When producing the ground truth annotations for this purpose each image goes through several steps in our annotation platform before it is delivered to a client. Using methods inspired by those outlined in ISO 26262 for developing safety requirements we take concerns like those above and break them down into similar requirements on our platform and processes in order to address them.
Consider the first hazard above, i.e. an object being annotated incorrectly. There could be several reasons for this, for example, the object being annotated when it shouldn’t and vice versa, or the object being annotated with incorrect properties. Using the functional safety approach we break down this hazard into a number of possible fault events in a fault tree analysis. In the end, basic failure of the resulting fault tree include events such as:
- An annotator made a genuine mistake
- An annotator did not know how the object should have been annotated
- The annotation task was unclear or insufficiently specified
- The annotation tool did not allow proper annotation of the incorrect object
This enables us to create requirements on our tools and processes to effectively mitigate or reduce the risk of basic failure events. This way of working with annotation hazards has resulted in us developing an onboarding process for training annotators on a specific task rather than just generic “box placement”, as well as a Guideline Agreement Process that ensures that Kognic, clients, and annotators all agree on what and how ground truth should be annotated.
This particular example is just one way in which we utilize this functional safety-inspired process to develop our platform. Other results include our quality assurance process, which you can read more about here, and our data coverage evaluation tools which are currently under development.
Reach out to us if you have any questions or want to discuss how we develop annotation tools to make safe perception possible!
Share this
Written by
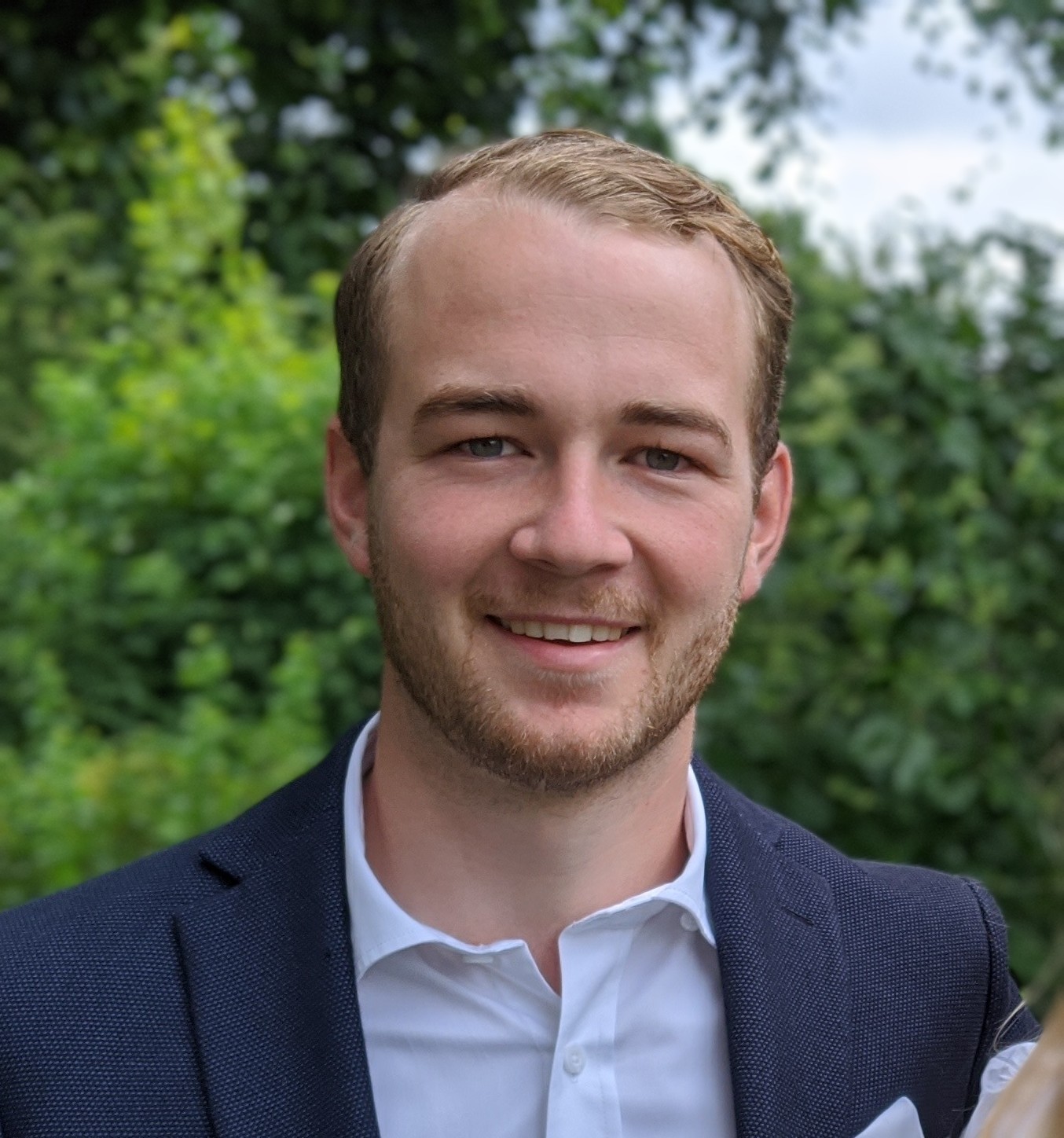
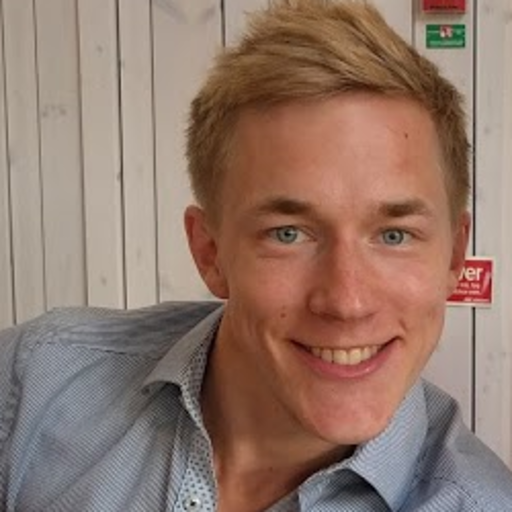