How can we create a great automated driving experience? On data, safety and accountability
How can we ensure a great automated driving experience without risks of accidents? While the race towards fully autonomous vehicles is ongoing, and with some self-driving vehicles already on the road, the actors of the automotive industry must guarantee their cars are safe in order to be moral leaders, minimize liability and strengthen their brand.
Lately, many media outlets are reporting and looking at Tesla’s cars due to safety matters. In this regard, the American National Highway Traffic Safety Administration (NSHTA) stated in May that since 2021 they had received 758 reports from Tesla owners who had experienced "phantom braking" (a random break activation when using Tesla’s Autopilot). Also in this line, according to a NSHTA report published a week ago, vehicles using Tesla's Autopilot software have been involved in 273 crashes during the last year, with 3 people dead. Nevertheless, and though this might seem a very recent issue, autonomous vehicles have been involved in crashes on public roads since at least 2014. And it is not just Tesla’s cars which have been causing "trouble". In November 2021, after a collision in California, Pony.ai lost its permit to test autonomous vehicles with driver. Moreover, Waymo vehicles have been involved in several crashes, even though in their 2020 report they state that their cars are rarely causing crashes but failing to avoid plausibly preventable crashes caused by human error.
Having said that, the NHTSA estimates that driver error is the main cause of between 94 and 96% of crashes in the USA. It’s us humans who get distracted, drive while drunk, miss traffic signs or react slower than we should. Under this perspective, autonomous vehicles still have the potential to reduce both the frequency and severity of crashes. But not just that: we believe that great automated driving experiences are possible, and that companies can achieve great customer satisfaction. But in order to achieve that, companies need a safe perception software that can train the models with high quality data, including as many scenarios as possible. A safe perception software that connects the interpretation of that high quality data with performance and safety.
A brief history of safety regulation in the automotive industry: ISO26262
In the automotive world there has been a long history of safety certifications. Even though there may be commercial factors involved, actors in the industry genuinely want to do good and, accordingly, they hire experts to minimize the risk of accidents.
As many know, there is a large amount of certifications available. The most famous one is ISO26262, which is a form of ISO certification specifically created for the automotive industry with the purpose of ensuring functional safety of components. This certification regulates the reliability required for a component and the methodologies by which it needs to be developed. Still, ISO26262 was thought for elements such as electronic components, wires, or breaks, where it’s easier to measure by applying external information to the component, verifying that it functionally responds in the expected way.
In the last decades, though, software has become a thing. And software is different to hardware in the sense that it changes faster and that it is more complex and harder to model, something that has made things very challenging for the automotive industry. Initially, software was only used for things like the infotainment system. However, in the last ten years, the concept of a software-defined vehicle has emerged, leading to more and more people in the industry talk about software-defined vehicles where the overall experience of using the car is to a very large degree determined by software, as opposed to interaction of electronic components that you can model.
Regulations, accountability and liability
If ISO26262 cannot be applied to self-driving cars, are there any regulations which help determine safety in software-defined vehicles? What happens with accountability in the event of an accident involving an autonomous, software-defined vehicle? At present, there's no regulation that explicitly tells the industry how to measure performance and safety of automated driving. Technology and machine learning have evolved so fast in the last years that we’re still in a regulatory limbo.
The things that exist today are the contracts buyers sign when they buy a car. In the absence of regulation, the industry is instead focusing on the concept of liability, that is, who is responsible and therefore blamed when an accident occurs. Being liable as a vendor means you’re contractually assuming some amount of responsibility if there's an accident when someone uses your product. If we continue with the example of Tesla, Tesla buyers assume no liability when signing the contract. That means that the company might never take responsibility if someone dies in traffic.
Nevertheless, even though buyers of Tesla cars agree to a contract with no liability, one could argue that the road hazard is a negative externality. This makes that the whole accountability question is still negotiated, partly in the consumer contracts with respect to liability, partly by the government issuing regulation, and partly by the OEMs’ own sense of responsibility. Practically speaking, in the case that a car is considered less safe we could have three of the following scenarios. In the first one, consumers would punish the company; in the second one, the government would step in; and in the third one, the company itself would start striving to build safer cars.
No shortcuts if we want zero accidents
There are no easy, fast and cheap shortcuts to what we like to call the space race of our time if what we want to achieve is safe self-driving and a great customer experience. The perception system will never exceed the examples and scenarios on which you trained it. That is why data, its quality and the correct interpretation of it is paramount.
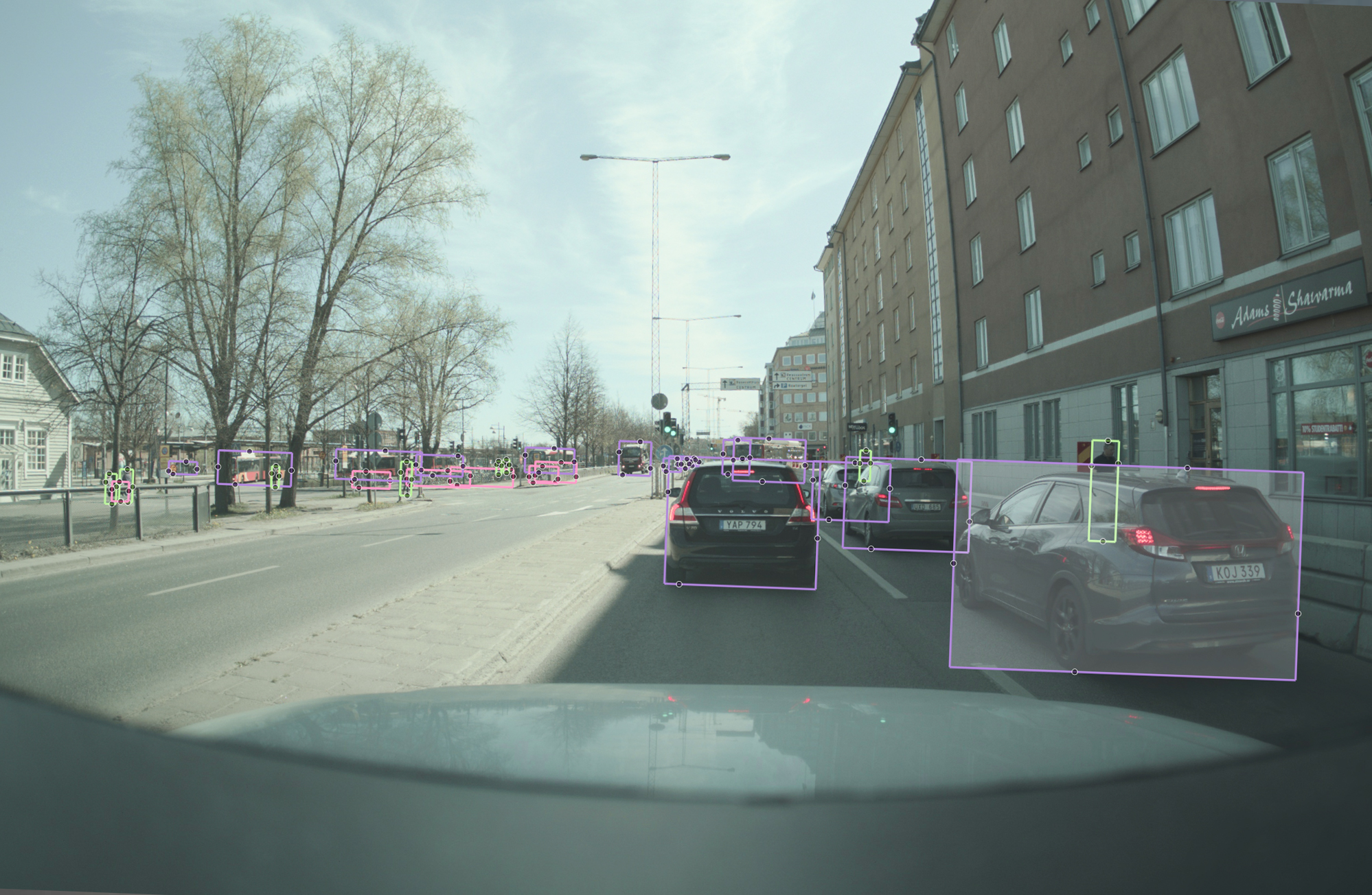
There is a true challenge connecting the interpretation of sensor readings with performance and safety. That, together with the challenge of handling a million of edge cases and the fact that things constantly change is why getting global coverage can only be at a very high cost. And this makes some actors in the industry revise their budget downwards and, thus, the safety of their cars. But the cost of the data should not be the main motivation if we want to develop safe perception systems. The main motivation to get safer cars in the market should be and is the interpretation of the necessary data for a great automated driving experience. This is also difficult as it poses an organizational challenge on how to delegate and balance perspectives across departments, as many teams depend on each other to achieve this goal and they can always suffer the risk of optimizing on self-interest.
Get in touch for a safe perception software you can trust
As a company, our purpose is to enable a great automated driving experience without accidents. Of course, that requires labeling data, but not for the sake of labeling. We label in order to improve performance and safety. Our customers depend on selling a system that people love using and that it is safe. And that cannot be achieved by just collecting tons of data, labeling it in the fastest and cheapest way possible, and without connecting the interpretation of it with performance and safety.
At Kognic, we create the connection between the interpretation of sensor readings, and performance and safety in the best and most ethical way possible. Because we only believe in a safe perception system that you can trust.
We know you care about safety. We also know that it is hard to balance the teams you have. Do you agree? If so, do you want to talk?
Share this
Written by
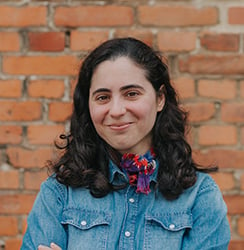