Graph Convolutional Networks for Complex Scenario Classification
A couple of weeks ago marked a significant milestone for us at Kognic as we celebrated the successful presentation of Tobias Hoek's master thesis. During his time with us, Tobias made impressive strides in the field of traffic scenario classification, contributing to its development and advancement.
In the automotive industry, scenario classification is vital for understanding complex driving situations. Tobias focused on developing a supervised scenario classification approach that accurately categorizes various scenarios, including the ego lane. He joined our team for his master thesis work, and his goal was clear: develop a cutting-edge approach to scenario classification.
Tobias' thesis utilized Graph Convolutional Networks (GCNs) for spatial aspects and Convolutional Neural Networks (CNNs) for temporal aspects, a potent combination that proved highly effective. As a result of it, his model achieved remarkable results, with a serious error rate of less than 30%, surpassing our expectations.
His work sets a benchmark for future research and refinement, offering new possibilities in traffic scenario classification. We're immensely proud of Tobias' contribution to Kognic and the broader field. Together, we'll continue to make our roads safer and more efficient.
Learn more about this research here.
Share this
Written by
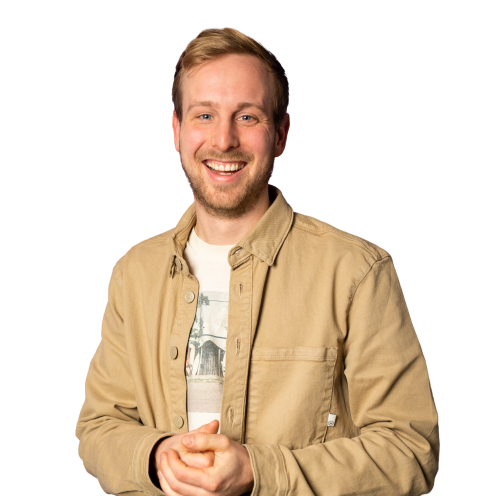