What challenges is automated driving currently facing… and what can we expect to happen in the coming years?
This won’t be the first or the last time we state that developing safe perception systems requires resources, time, skilled technical teams and the right tooling. As we said in our article Obstacles you can encounter when working with your model - and what to do about them, the challenge isn't just building a model for your system, but building an integrated ML system that works.
Data science and machine learning are advancing in giant steps, and it might be that many of the pitfalls technical teams meet when developing their models become a thing of the past in a few years. Either way, what we know is that a correct ODD with the right specifications, representative data coverage, and enough data of the highest quality can facilitate the road towards great automated driving experiences. Because this is how you teach the machine to react appropriately in a wide range of situations, including dealing with new ones, and uncertainties involving pedestrian behavior, random objects, and different road settings.
It’s understandable that this technology must be as accurate as possible in order to be safe and reliable, and thus be trusted by society. Until that moment, there are some challenges that the industry needs to address so that automated vehicles become the norm, little by little. We address those in the following paragraphs.
Challenges that will be a matter of the past in the near future
1. The ability to appropriately respond in any situation
One of the biggest challenges we are facing in the industry is teaching the machine how to suitably respond in any type of situation that the vehicle meets.
As a safety-critical application, ensuring the safety of automated technology is vital. However, as of today, it is impossible to teach a model all cases and scenarios in the world, since it is not viable to make an exhaustive list that includes all situations, including rare ones that represent critical situations or edge cases. What is currently feasible is to make sure there’s as much data coverage as possible, and to be able to explain that the model’s decisions are appropriate. By doing so, we make sure that we train our machine learning models with enough cases with a wide variety of scenarios, so that dangerous situations and uncertainties are reduced dramatically.
How do we know if we have enough data? It is not as simple as it may sound, and we have entire teams who deal with data coverage matters on a daily basis. In this regard, describing the training data accurately with guidelines and an edge case description as representative as possible can make a big difference. Then, once the description is transferred to the model, we should expect the model to capture the desired coverage. We would know what the model wouldn’t cover, but that would let teams select and add the additional data needed in order to complete the picture.
We’ve mentioned explainability as a factor that can help solve this coverage challenge. Explanations can be of help in different scenarios for various reasons. Some of them are mentioned in Explainability of deep vision-based autonomous driving systems: Review and challenges (Zablocki et al 2022, 1):
“When the system works poorly, explanations can help engineers and researchers to improve future versions by gaining more information on corner cases, pitfalls, and potential failure modes (Tian et al, 2018; Hecker et al, 2020). Moreover, when the system’s performance matches human performance, explanations are needed to increase users’ trust and enable the adoption of this technology (Lee and Moray, 1992; Choi and Ji, 2015; Shen et al, 2020; Zhang et al, 2020). In the future, if self-driving models largely outperform humans, produced explanations could be used to teach humans to better drive and to make better decisions with machine teaching (Mac Aodha et al, 2018).”
In short, explanations let the public better understand how these vehicles work and, therefore, this increases their trust towards them. This also has the potential to help improve the way humans drive.
2. Facing ethical dilemmas
Increasingly automated vehicles will have to make decisions very quickly, and some argue that these vehicles might face situations where they must make moral decisions. How can we make sure that, for instance, in case of an imminent accident, the car makes the right decision? What even is the right decision?
The ultra-famous trolley problem (what is the most ethical thing to do in case of an accident? Could be, for instance, to save a pedestrian, or to save the driver?) can be interesting when talking about dilemmas in automated driving. The discussion around it highlights what the moral decisions are, and to what extent technical products can be held responsible for such decisions.. Nevertheless, although the trolley problem might lead to interesting conversations, which can be valuable when developing products for safe perception, a machine trained with math is not moral. And rather, the most realistic and helpful point to discuss here would be how responsibility is to be handled in case of unexpected outcomes.
In our article Navigating some ethical dilemmas of increasingly automated driving, we talked about three main ethical dilemmas that are driving an essential conversation in our field: the trade-off between precision and recall; accountability; and bias and fairness. Their common denominator and most important conclusion to draw was that it is paramount to put a great deal of effort into data coverage. This pays off in the later stages of the model development and, ultimately, in the safety and performance of the perception system.
As we have explained other times on this blog, selecting the data that actually matters for your safety case and providing the right description of your domain is the best starting point when addressing the data coverage question. In this regard, we help our customers to identify and communicate gaps in coverage connected to the domain specific ODD that need to be filled, enabling data collection prioritization of scenarios that impact performance and safety the most. And that’s how continuously monitoring data distributions via our Data Coverage Improvements module ensures that teams get the data they actually need.
3. Lack of regulations
There is still a significant gap between the current regulations and increasingly automated and autonomous driving technologies. Although more organizations and countries are starting to write recommendations and even beginning to legislate in this matter, the question of what is safe enough and how to measure it is still an open question. Besides, even if these questions are answered, how would you be able to argue that you have fulfilled them? Coverage will play an important role.
There will be a need for unified regulations. In the case of Europe, it would make sense that these laws are applicable in every member country to avoid confusion and ensure safety. Regulations are also needed in order to address the accountability question, which we talked about in How can we create a great automated driving experience? On data, safety and accountability and Navigating some ethical dilemmas of increasingly automated driving. As we commented there, if ISO26262 cannot be applied to increasingly automated technologies, what happens with accountability in the event of an accident involving a software-defined vehicle? As there's no current regulation that explicitly tells the industry how to proceed or measure automated driving performance and safety, the only thing that exists today are contracts when buying a vehicle. Therefore, at the moment the focus is set on the concept of liability: who is responsible and therefore to blame when an accident occurs. In most cases, it is the manufacturer. However, in the case of Tesla, the responsibility lies entirely with the driver.
4. Data Coverage
At this point, we could describe having sufficient data coverage as the crown jewel. It is present in most of our articles and we’ve also talked about it above. Data coverage knowledge will not only increase trust in your performance, but it will also help to reduce your development and verification time. Having said that, ensuring enough coverage is a difficult task per se and it rarely comes without struggles. The main four challenges teams face when defining the coverage are ensuring that the data coverage is representative (How to ensure that data accurately reflect the ODD?), comprehensive (How to ensure that the entire ODD is covered?), it tackles long tail scenarios (How to discover the long tail scenarios in the ODD?), and that it is sufficient (How to ensure that calculated metrics are statistically significant?).
Conclusion
Having talked about these challenges, automated driving technologies have the potential to be a force of good. To begin with, they can drastically reduce accidents, as well as environmental pollution by up to 60% according to Ching-Yao Chan’s article Advancements, prospects, and impacts of automated driving systems. What’s more, and as exhaustively listed in this article (Chan 2017, 211-212), automated driving technologies can bring a more efficient mode of transportation; better managed traffic flows; more efficient and better planned infrastructure; greater mobility freedom for the disabled, and senior, amongst others; and enhanced safety, reliability, security, and productivity.
It is only a matter of time and advances in technology. What is now thought to be a challenge will no longer be an issue. We think this will happen sooner rather than later. Until this day comes, we will keep working behind the scenes to help our partners get their perception systems ready, using the most sophisticated tools available. 💪
Share this
Written by
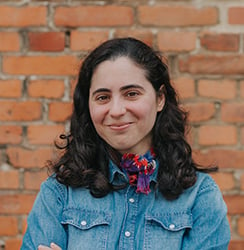
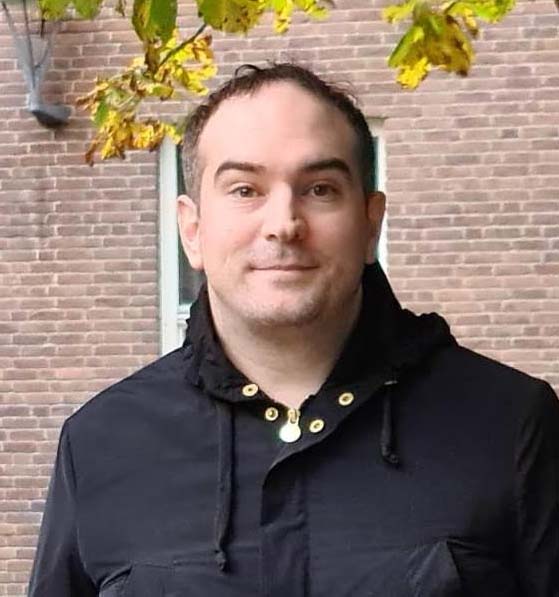
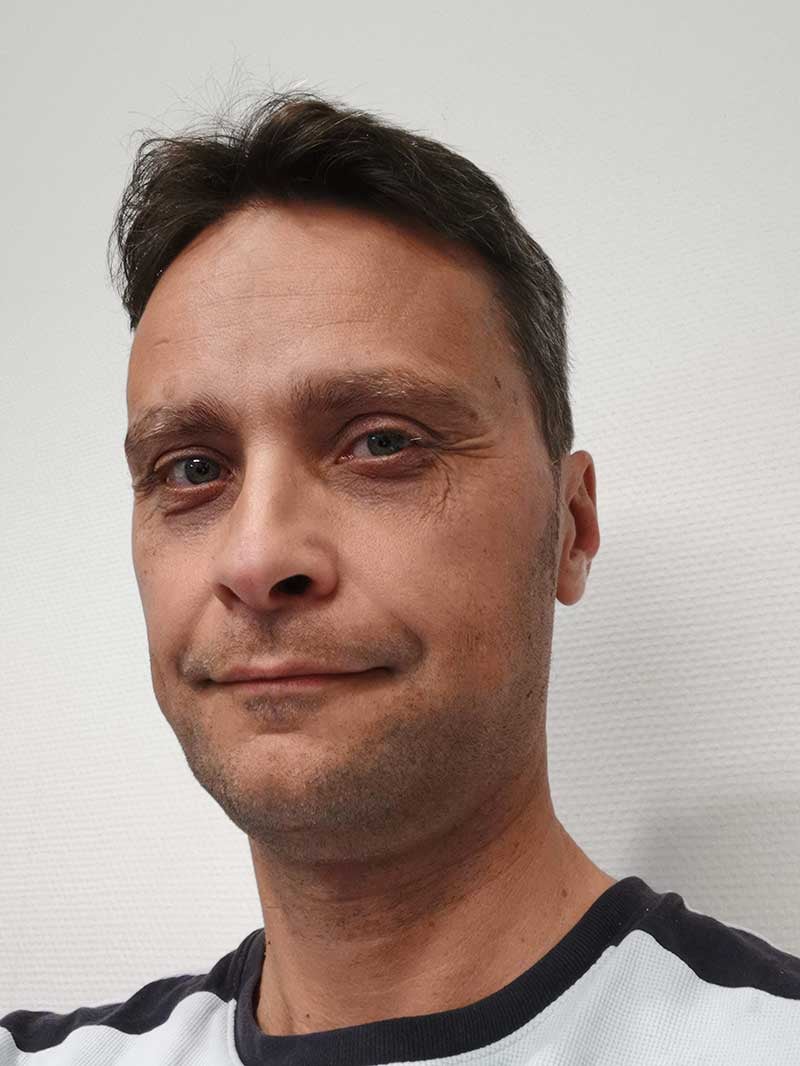