Navigating some ethical dilemmas of increasingly automated driving
Technological breakthroughs typically generate debates around social and ethical issues. Increasingly automated driving is no exception, and researchers have been raising different issues in scientific papers and the media for a number of years.
In this piece, we will present three ethical dilemmas that are driving a fundamental conversation in our field. In addition, we will provide answers on how we’re approaching these ethical matters when working with safe perception at Kognic. These issues are important not only for increasingly automated driving, but for any field within AI.
The trade-off between precision and recall - an ethical dilemma?
Which is worse? A false negative or a false positive? This is a question our customers ask us and we ask ourselves frequently. What would be the safest and most ethical solution? Let us explain this with an example involving mannequins and humans.
Imagine a scene in the street where there are mannequins as well as people. For safety’s sake, you might argue that you’d rather have mannequins labeled as pedestrians, than pedestrians labeled as mannequins. In this way, you decrease the risk of accidentally thinking that a pedestrian is a mannequin. In an emergency situation, you may need to hit the mannequin to save the lives of actual pedestrians.
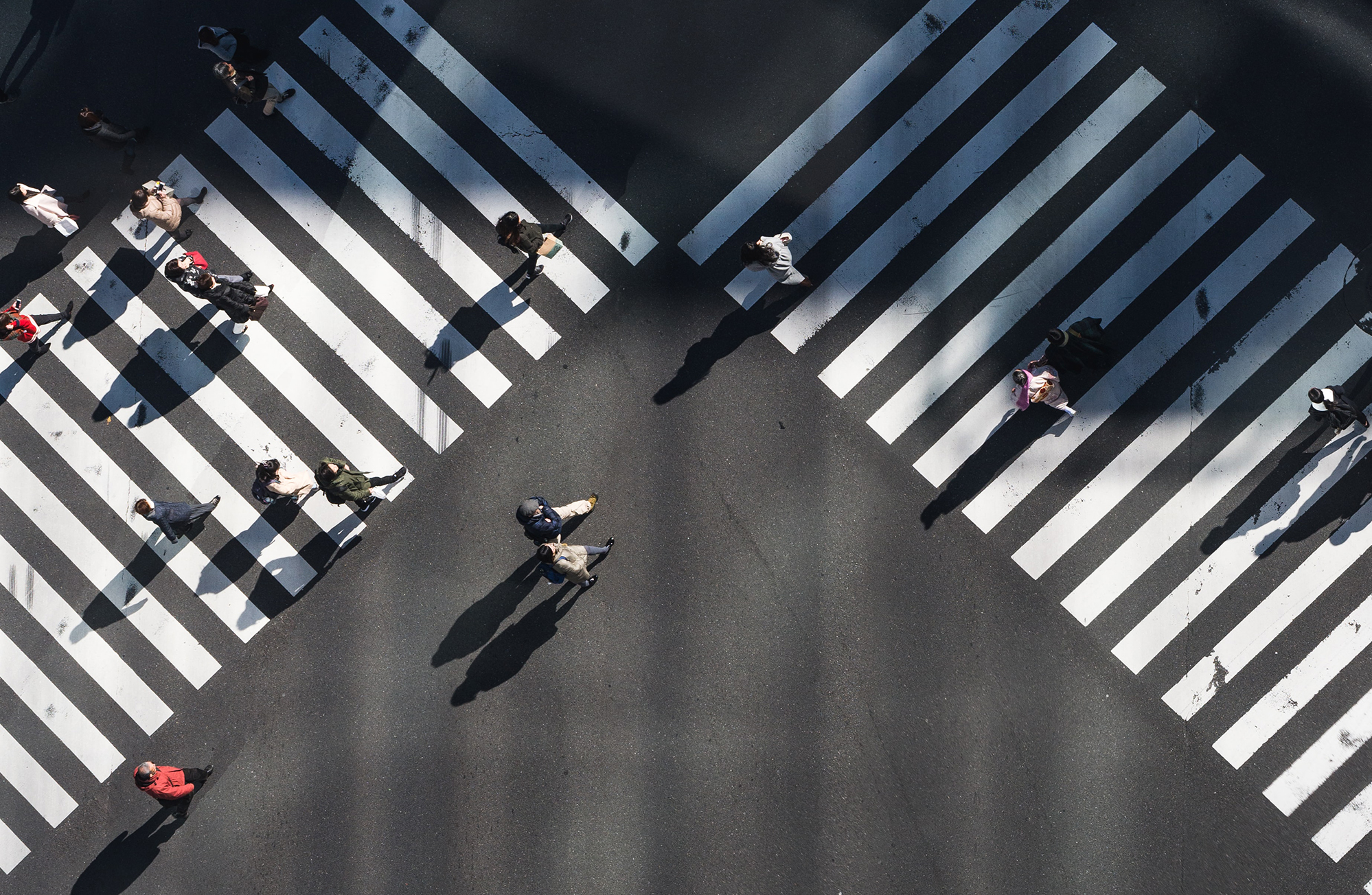
Navigating the classic trade-off between precision and recall is something that we need to do for the model and data used for training and evaluation. What to optimize the perception system for depends on function, level of autonomy, context and object type. In some cases, it’s very clear which error needs to be avoided at all costs. However, in some cases, the trade-off is much trickier. By digging into the data and understanding the various situations captured, we help clients to navigate the trade-off and get data that reflects their needs.
Accountability
In order to ensure safety and accountability, there is a need for common regulations. Even though we see an increasing number of recommendations and legislations being made by organizations and countries, there is still a significant gap between current regulations and autonomous driving technologies.
In the field of ADAS and AS, in the case of an accident or a car not meeting certain safety expectations on the road, someone must be held responsible. We have already shared some of our thoughts on this topic in our blog article entitled, How can we create a great automated driving experience? On data, safety and accountability. In this article we discussed examples of accidents involving Tesla and Waymo cars. As we commented there, if ISO26262 cannot be applied to increasingly automated technologies, what happens with accountability in the event of an accident involving a software-defined vehicle? Currently, there is no regulation that explicitly tells the industry how to proceed here or how to measure the performance and safety of automated driving. The focus is therefore on the concept of liability: who is responsible and therefore to be blamed when an accident occurs? In some cases, the manufacturer is the one to be blamed. However in the case of Tesla, the responsibility lies entirely with the driver.
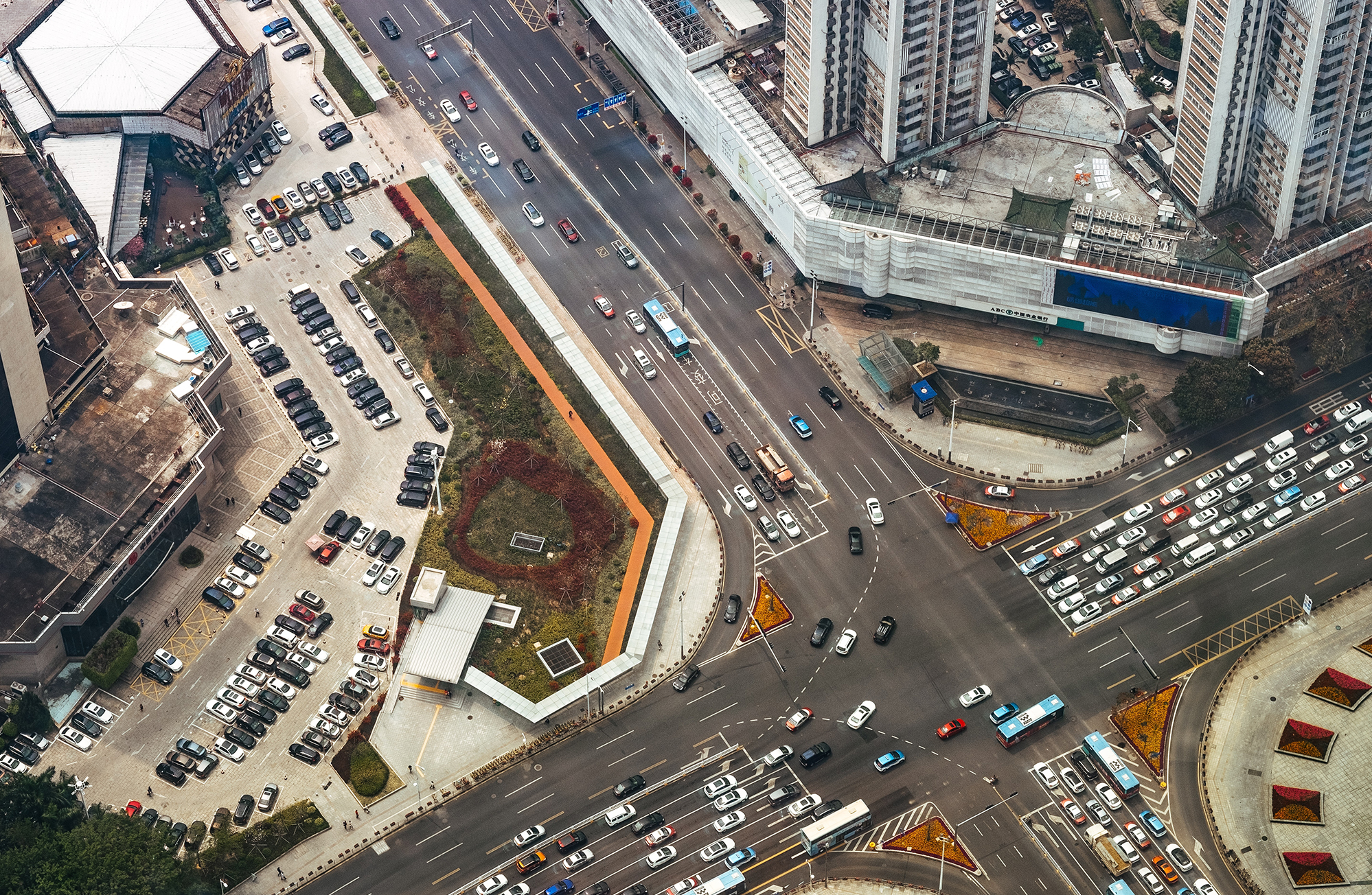
Our team of Perception Experts keep up to date with the latest developments in terms of regulation and standardization, and they are also members of organizations such as ASAM. As a result, they are knowledgeable of the latest advancements in this matter. When helping our customers create suitable annotation guidelines, they take these trends into account and set safe performance expectations for each use case.
Bias and fairness
How can a more automated future be as fair as possible? Research has shown that algorithms can also be biased. One of the most renowned works in this field is Gender Shades: Intersectional Accuracy Disparities in Commercial Gender Classification (Buolamwini, 2018). In this work, Buolamwini showed how machine learning algorithms discriminate based on classes like race and gender. There are accuracy differences in terms of facial recognition between male and female, and between lighter and darker skin types. The Fitzpatrick Skin Type classification shows that darker-skinned females are the most misclassified group (with error rates of up to 34.7%) (Buolamwini 2018, 1). In the case of increasingly automated driving, this could lead to safety issues. AI researchers, regulators and human rights organisations are rightly concerned over models reproducing discrimination which affects both people’s rights and safety.
This issue is linked to data coverage, something we’re very good at! As we’ve described in our blog previously, selecting the data that actually matters for your safety case, and providing the right description of your domain, is a good starting point for data coverage. In this regard, we help our customers to identify and communicate gaps in coverage that need to be filled in connection to their domain specific ODD, enabling data collection prioritization of scenarios that impact performance and safety the most. Continuously monitoring data distribution via our Data Coverage Improvements module ensures that teams get the data that they actually necessitate.
Therefore, there is a clear need for all actors in the industry to ensure sufficient data coverage. Here we are discussing providing enough examples of people of different genders, and races, so data can be classified with minimal errors and at the required safety level. If you’d like to know more about how our software can help here, we recommend that you read this piece that we wrote recently.
Following ethical principles will always be the right choice
There’s a raison d’être for our continuous efforts towards a more ethical way of working. No matter if it’s about buying data, training models or deploying autonomous functions in the real world, ethics need to be at the heart of what we and other actors in the automotive industry do.
We’ve mentioned concepts in this article such as data coverage, liability, and fairness. Although there are still no answers to some of the questions raised here, it is positive to continue the conversation and also remember what we should do. Because what we do now and how we do it will shape our future. The common denominator here is to put a great deal of effort into data coverage. This will pay off in the later stages of your model and ultimately in the safety and performance of your perception system.
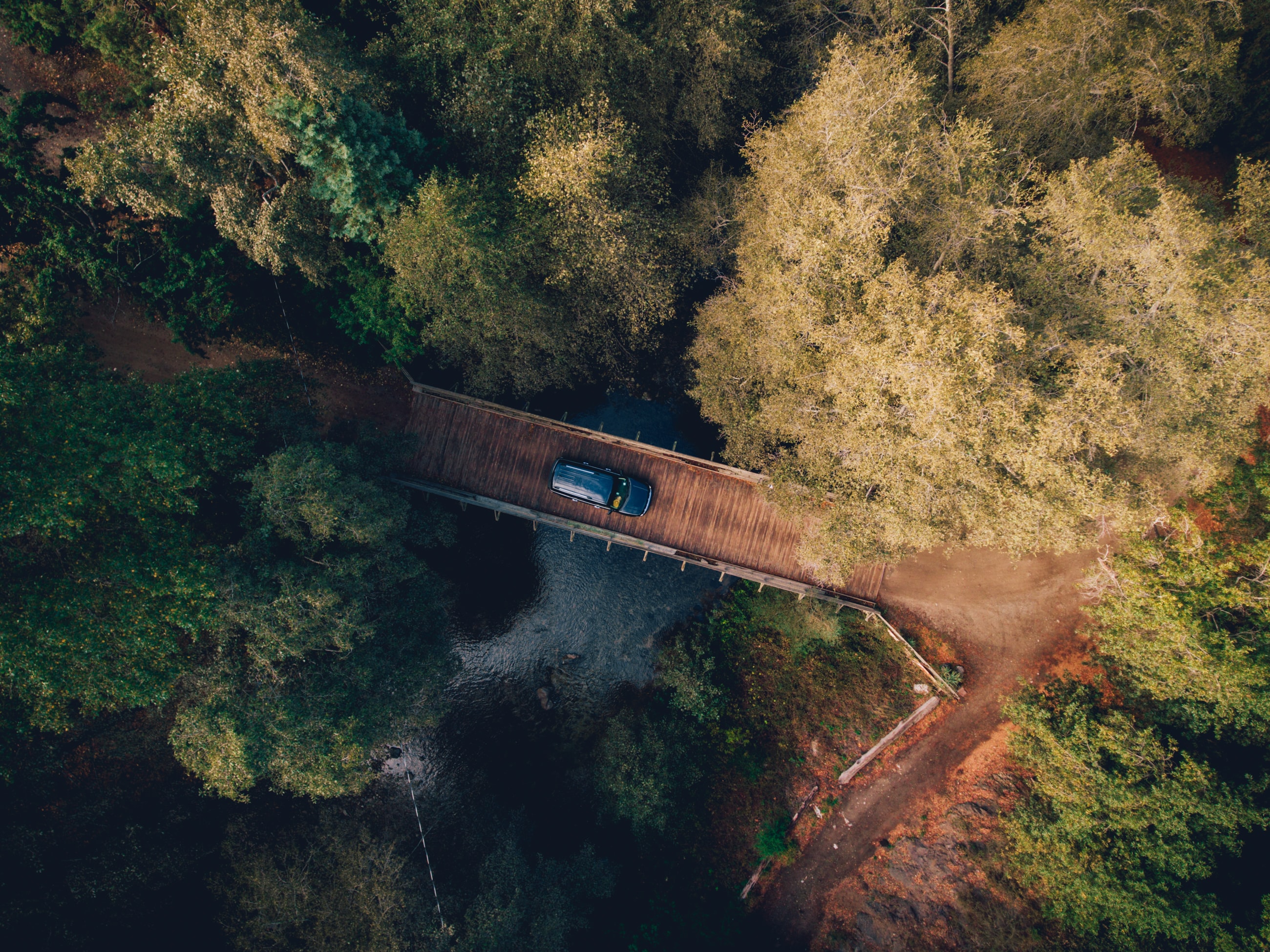
Share this
Written by
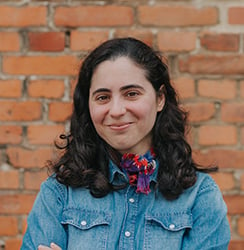