The Evolution and Future of Autonomous Driving Technology: An Interview with Daniel Langkilde, CEO of Kognic
The emergence of autonomous driving has undoubtedly marked a paradigm shift in the automotive industry. However, years of research and billions of dollars have been spent on getting cars to drive themselves, and we're still learning. In this blog post, we'll explore the current state of autonomous driving, its challenges, and the potential future of the technology based on an interview with our CEO, Daniel Langkilde.
The Current Approach to Autonomy
According to Daniel, the current approach to autonomous driving breaks down a very complex problem into its components—perception, prediction, and planning. Each of these is a considerable challenge in its own right, and the sequential process can lead to information loss.
In his own words, "The trend lately has been that people feel we are sort of throwing away too much information doing this as sequential steps. And instead, some argue we should try and solve this using one giant neural network, which is sort of known as an end-to-end model or a fully differentiable stack."
The Role of 'World Models'
One of the key components of this approach is what Daniel referred to as the 'world model,' or the common sense that a machine must be equipped with to make sense of the complex world around it. However, creating a world model for machines that can accurately predict the future based on previous frames is a significant challenge.
Daniel discussed Tesla's approach to this, mentioning that "Tesla, obviously also should be included, that are trying to train very large quote-unquote world models based on observing large quantities of driving." However, he also noted that this is a relatively new approach, and it's still not clear if it works.
Looking Towards the Future
When asked about the future of autonomous driving technology, Daniel highlighted that there are many ways in which autonomous vehicles can be tripped up, and expanding the operational design domain for these vehicles still looks to be a challenging and expensive task.
He stated, "Machine learning still struggles to build sort of common sense and generalize to new situations. That's our biggest challenge dealing with all that ambiguity."
Daniel also highlighted the importance of being open to new paradigms and approaches. He said, "If there's evidence that there's a new paradigm, all it takes is a few like bold entrepreneurs. And then it doesn't matter if existing companies hold on to their old ideas. Someone else is going to try the new one and maybe it works, maybe it doesn't."
In conclusion, while autonomous driving technology has come a long way, there are still significant challenges to overcome. By continuing to innovate and explore new approaches to autonomy, we can hopefully move towards a future where self-driving cars become a safe and reliable reality.
Listen to the full interview here.
Share this
Written by
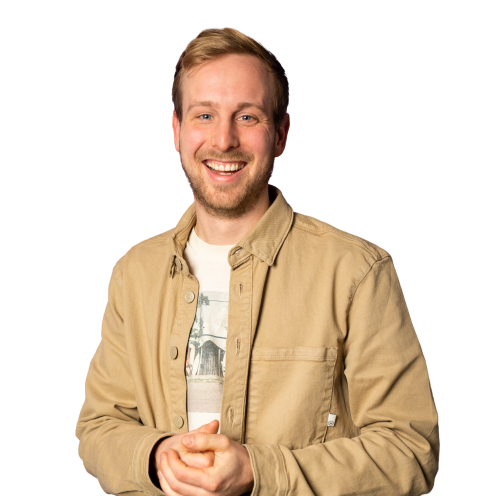