Trends in Perception within The Automotive Industry
In a recent webinar, Olof Wahlström presented some fascinating insights into the trends in perception within the automotive industry. As machine learning continues to advance, we aim to accelerate this progress by focusing on sensor fusion, particularly in the automotive sector. Olof emphasizes that the key to achieving optimal performance lies in datasets, which currently pose challenges for machine learning engineers.
Multiplication of Sensors
One of the major trends in perception is the multiplication of sensors. As tasks become more complex and autonomous, there is a growing need for a wider variety of sensors to ensure accurate perception of the world. LiDARs, cameras, radar, and other sensors are being combined in new and innovative ways to capture a more comprehensive view of the surroundings.
Continuous Improvement of Sensors
Another notable trend is the continuous improvement of sensors. Cameras now offer higher resolutions, while LiDARs can detect objects over long distances, even up to 400 meters or beyond. The emergence of visual radar technology also adds to the advancements in sensor capabilities. The reason for utilizing multiple sensors lies in their individual strengths and the need to complement each other under different conditions. For example, in challenging weather conditions, cameras may struggle in darkness, highlighting the importance of incorporating other sensor types.
Diversification of Testing and Validation Scenarios
The types of scenarios being used for testing and validation are also diversifying. To make these functions viable on the road, ODDs tend to be more focused and specific. Different levels of autonomy, ranging from L1 to L5, are being explored. While L4 and L5 levels are predominantly seen in robot taxis operating in urban areas, traditional OEMs have concentrated on lower levels of autonomy like L3, with a greater emphasis on highway driving. However, there is a growing trend towards expanding the ODD to include more urban driving and moving towards L4 autonomy.
Evolution from Static to Sequence-Based Approach
Perception is evolving from a static to a sequence-based approach. The temporal aspect has always been critical, but there is now a shift towards incorporating the temporal dimension directly into machine learning models. This evolution has implications for the data used in training these models.
Leveraging Larger Datasets for Higher Performance
As datasets grow larger, advancements in machine learning algorithms and techniques are being leveraged to achieve higher performance. The automotive industry is no exception to this trend, although the growth in datasets is driven by multiple factors, such as the increasing number of sensors and expanding ODDs.
Ethical Considerations
Ethics play a crucial role in this domain. Collecting and processing large amounts of data requires significant labor. Unfortunately, the industry still faces challenges related to poor working conditions, low work stability, and unfair wages. It is vital for both buyers and sellers in the industry to take social responsibility seriously and ensure ethical practices are upheld.
We have incorporated these trends into our tools and processes. In our next blog post, we will be discussing in depth how we have adapted.
Share this
Written by
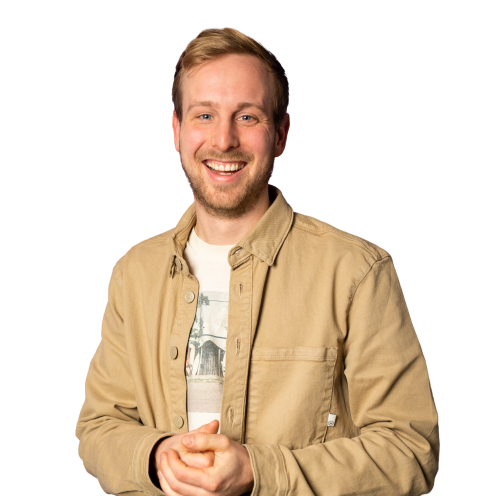